Module 3 Chapter 6: Study Participants
Research informing our understanding of diverse populations, social work problems, and social phenomena is entirely dependent on the participants who provide the data and observations for analysis. Guidelines for engaging participants vary by research approach: qualitative, quantitative, or mixed-methods, as well as by study purpose (descriptive, exploratory, or explanatory questions). In this chapter you will learn about:
- Sample size implications
- Strategies for establishing study samples
- Participant recruitment
- Participant retention
- IRB concerns for research involving human participants
What Is a Sample?
First things first: understanding this chapter requires an understanding of what a sample is. In research, a sample is a group drawn from a population for the purposes of observation or measurement. If we measured an entire population, we would not be talking about a sample. It is usually unreasonable to attempt this feat of measuring an entire population to answer a research question—although it is sometimes possible in evaluating a small-sized program. Ideally, the sample drawn is a good representation of the population. This allows an investigator to offer generalizations based on the study results derived from the sample. Many of the quantitative statistics used in research are based on certain assumptions about the relationship of the sample to the population. If sampling error is small, then statistical values observed for the sample will be a good representation of the statistical values we would see for the entire population if we could really measure them all.
A note about populations is warranted here: a research population is not the same as the entire population. A research population is defined by certain variables of interest in the study being conducted. For example, consider a study of service needs experienced by adults following release from incarceration (Begun, Early, Hodge, 2016). The population relevant to this study was defined as men and women preparing for release from jail or (state) prison—it was not all people, or even all incarcerated people (not juveniles, not persons in federal prison, not individuals who are still serving sentences). The results of the study are generalizable only to this specific population, and since the data were collected only in the state of Ohio, generalizations to other states are made with caution (see Figure 6-1).
Figure 6-1. Sphere of generalizability to study population
Matters of Sample Size and Diversity
The question of how many study participants are needed for a specific study is complicated to answer. First, it depends on the study approach and aims. Most qualitative research is not intended to be generalizable to a larger population, but most quantitative research is. If the aim of a qualitative study is to develop a deep, rich understanding of a construct, event, experience, or other phenomenon, then the number of study participants needed might be relatively few. For example, in grounded theory studies, the need is to get to a point where all or most facets of the topic are addressed and where enough responses cluster together to have a picture of common themes emerge from the data. This ideal point of saturation is achieved when new ideas, not expressed by previous participants are no longer being presented by subsequent participants. The recommended number is in the 20-30 participant range (Creswell & Poth, 2018). Similarly, in ethnography, the number of participants depends on how many observations are required to achieve a clear picture of the culture-sharing group (Creswell & Poth, 2018). In narrative studies the aim is to develop the story of a single individual, or a few individuals involved in a single event. In focus group studies, the emphasis is on group process, so the recommendation is 5-10 persons per group—the number of groups varies from 1 to several.
The aim of quantitative research lies in sharp contrast: the aim is to generate information that is generalizable from the sample to the population. Therefore, the numbers needed are usually greater for studies designed around quantitative than around qualitative approaches. Table 6-1 presents an example generated from 12 social work students using samples of M&Ms® candies to estimate the proportion of green M&Ms® in the population of M&Ms® candies (adapted from Begun, Berger, & Otto-Salaj, 2018, p. 8).
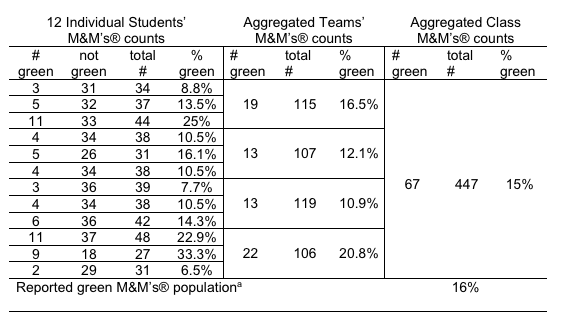
As you can see, when the individual students’ sample sizes were between 18 to 37, the range of values for proportion (%) of green candies was wide—as low as 6.5% and as high as 33.3%. As students combined their samples (teams of 3), the sample sizes from 106 to 119 had much less variability in the observed proportions: 10.9% to 20.8%. Finally, when the teams’ samples were combined into one single class sample of 447 candies, the sample’s observed proportion of 15% was close to the actual proportion for the population reported by the company (16%). (Yes, they ate the candy after completing the demonstration.)
Second, the diversity of study participants is at least as important as numbers in qualitative research studies. To ensure rich coverage of a topic, investigators seek participants with differences likely to be important to that topic—an approach that maximizes variation among study participants so that different perspectives will arise in the data. The approach to selecting participants is purposeful. For example, in a grounded theory qualitative study of benefits experienced by older adults in adult day services programs, investigators interviewed 28 service recipients (Dabelko-Schoeny & King, 2010). More important than the numbers, however, is that the investigators intentionally, strategically engaged participants from 4 different programs. The group of study participants ended up being Caucasian and African American women and men, differing in marital status, ranging in age around a mean of 78 years, experiencing different types and numbers of medical conditions, and ranging in income ($0-$88,000 annual income). The investigators’ conclusions were that five themes expressed by the study participants fit into two general categories: program experiences and perceived impact of experiences. The program experience themes included social connections with other participants, empowering relationships with staff, and participation/enjoyment of activities and services. The perceived impact of experiences themes included perceived improvement in psychosocial well-being and perceived decrease in dependence and burden on their primary caregiver. These results contribute to an understanding of the perceived benefits experienced by persons who participate in adult day services programs.
Just as sample diversity is important in qualitative research, the issue of sample heterogeneity is an important consideration in quantitative research. The challenge played out in quantitative research concerns the sample having diversity that is representative of the population to which results will be generalized. In contrast to the purposeful selection process used in qualitative research, strategies for randomly selecting participants into quantitative studies are more common. More about this topic is presented in the next two sections.
Filling a Quantitative Study Design
Let’s revisit a study design example from Chapter 4—the 2 X 3 design with empty cells. The original example was about waiting to see how individuals sorted themselves out in terms of the two variables of interest. This time, we are going to use the diagram to help figure out how many people of each type investigators need to include in our sample for a quantitative study. Imagine that a planned study is about participants’ ratings of an outcome variable—maybe likelihood of engaging in additional college education—and the investigators wish to determine how age group and employment status relate to this outcome variable. The statistical analyses planned by the investigators require a minimum of 10 persons in each cell (more about this in Module 4).
The result would be the need for ending up with a minimum of 60 study participants, recruited from each of the 6 types (10 x 6=60).
Employment Status | ||||
---|---|---|---|---|
Age Group |
Fully Employed | Partially Employed | Unemployed | |
<30 | 10 | 10 | 10 | |
≥30 | 10 | 10 | 10 |
Now imagine that the investigators, based on their review of literature, have decided that they really need 3 age groups: 18-25, 26-35, and over 35. Let’s see what this does to the design and number of participants needed. As you can see, we now have a 3 X 3 design, which makes 9 cells. With 10 participants needed per cell, the minimum sample size is now increased to 90.
Employment Status | ||||
---|---|---|---|---|
Age Group |
Fully Employed | Partially Employed | Unemployed | |
18-25 | 10 | 10 | 10 | |
26-35 | 10 | 10 | 10 | |
>35 | 10 | 10 | 10 |
Thus, one answer to the question of how many study participants are needed in a quantitative study is that it depends on a combination of the study design and the intended statistical analysis requirements. In our next course, SWK 3402, you will also learn about matters of statistical power and effect size in determining how many participants are needed in an experimental study about interventions. For now, it is sufficient to understand that the more complex a study design, the greater the number of study participants are required.
Sampling Strategies: Probability and Nonprobability
Earlier, when discussing the strategy used for generating the sample in a qualitative study we used the word “purposeful.” This means that selection of study participants was not left to chance. In quantitative studies, however, investigators need to minimize the extent to which bias enters into the participant selection process if the sample drawn from the population is to be reflective of that population. They need every individual in the population to have the same chance, or probability, of being selected. This means using random selection strategies.
Random Selection. Random selection refers to strategies used to recruit participants into a quantitative study with the aim of maximizing external validity (the extent to which results from the sample can be generalized to the larger population). Random selection is considered a probability sampling strategy since the probability for each and every member of a population to be included in the sample is the same. This use of the word “random” should not be confused with the concept of random assignment in an experimental study. Random assignment refers to dividing the randomly selected sample into assigned experimental groups—like who receives the experimental, new intervention being tested and who receives the usual form of intervention (a lot more about this in our next course, SWK 3402).
Random selection is never perfect—there is always some error in the degree to which a sample represents a population. The aim is to minimize this error, especially to minimize systematic error or bias in the sample. Simple random sampling begins with a known pool of possible participants: they are the sampling frame. The investigator is going to select a specific number of those possible participants, at random, so that each possible participant has the same probability of being selected as any other participant. For example, imagine that a social worker wants to use the residential zipcodes for a randomly selected group of individuals in a program for men and women court-ordered to receive treatment to stop their intimate partner violence behaviors to determine the best locations to place a new branch of the program. The social worker decided that 20% of the zipcodes should be utilized. The sampling frame, or available pool, is all those served over the past year—for this example, let’s say it was 1,200 persons. This means that the need is to select 240 participants for the study (1,200 x .20=240). The social worker in this situation could assign each “case” in the program a number from 1 to 1200. Then, a statistical program could be used to randomly select 240 of those numbers. An example is the free random number generator called Stat Trek (https://stattrek.com). The social worker enters 240 in the box for how many random numbers are desired, 1 for the minimum value, 1200 for the maximum value, and does not allow duplicate entries (“false” means that once a number has been selected it is removed from the pool so every number generated is unique.) The resulting table tells the social worker to use the zipcodes from “cases” numbered 186, 251, 58, 635 and so forth to the last one, 246.
Essentially, this is like drawing numbers from a hat or generating lottery numbers on television. Unfortunately, sometimes the size of the starting pool is unknown or changes. For example, a social worker may want to obtain client satisfaction information from 10% of families served by an agency. There is no pre-existing pool to draw from, the need is for 10% of whoever comes into the program moving forward in time. In this case, investigators need to rely on systematic random sampling strategies—retaining an element of random selection, but more vulnerable to systematic bias. For example, an investigator might decide to collect mental health and substance use screening data from every 10thperson who comes to the emergency department for care. Assuming that there exists no systematic bias in the pattern by which people come to the emergency department, this plan is effective. Where bias enters the picture is if the sample selection is conducted only during “office hours” (M-F, 8-5). The people who come in on weekends and late night may be very different than those who are sampled.
As a different example, for a study about family recreational time, taking every 10th child enrolled in after school programs is fraught with potential bias problems. First, families with more than one child in the programs have a greater chance than single-child families of being selected. Second, this type of list is already systematically arranged from the sequencing of when children are enrolled—there may be some systematic differences between those who enroll early and those who enroll later. Working from alphabetical listings has a similar problem: certain last names are more common than others in the U.S.—Smith, Johnson, Williams, and Brown are the top 4. This alters the chances of the persons with names in that part of the alphabet. Sampling bias is potentially introduced when the pool from which selection is made is systematically arranged.
Convenience Sampling. Probability sampling through random selection is not always essential because sometimes the research aims do not include generalizability back to the population. An example of this scenario is a pilot or feasibility study being conducted in preparation for a larger scale study with external validity being addressed. Another example is a study where it simply is not feasible to draw a representative sample from the general population of interest. Investigators might rely on selection from among those most easily accessed—called convenience sampling. In these instances, potential bias from non-probability sampling is recognized as a generalizability limitation in interpreting the results.
For example, consider the comparative, exploratory study conducted with social work students in 4 countries: United States, Greece, Cyprus, and Jordan (Kokaliari, Roy, Panagiotopoulos, & Al-Makhamreh, 2017). The purpose of the study was to extend our understanding of social workers’ perceptions concerning non-suicidal self-injury. The selection of these countries was intended to maximize heterogeneity (diversity) in terms of cultural values and religious belief systems: inclusion of students with Christian, Greek Orthodox, and Muslim religious backgrounds, and nations differing in social/political orientation toward individual and women’s rights. The students participating in the survey study were those easily accessible to the investigators who were associated with social work programs in those countries. The investigators identified differences and similarities in the students’ responses which are suggestive of the need to better address the problem of non-suicidal self-injury in social work education; however, they also identified the potential limitations of the study related to the convenience sampling approach. Student surveys may not be a good representation of social work practitioners, either. The observed differences in this study were that Jordanian students viewed the problem behaviors as being associated with weak/absent ties to religion, while U.S. and Greek/Cypriot students attributed the problem to mental illness and socioeconomic factors. Students from each nation were equally unprepared to understand treatment of the problem. One concern with how to better educate social workers about non-suicidal self-injury is that the behaviors may differ in the varied cultures/countries, and marked differences in stigma exist, as well.
Snowball Sampling. Social work investigators are often asking research questions related to difficult-to-locate populations—questions about uncommon problems, stigmatized phenomena, and intersectionality issues. Quite possibly, individuals who meet the study criteria know others like themselves. In these cases, investigators may rely on snowball sampling strategies: the sample builds itself from a few core individuals who identify other participants, and they identify still others. The name comes from how you might build the base of a snowman: start with a small snowball, roll it in the snow and as more snow adheres to it, the snowball grows larger (assuming it is good packing snow). This is another form of non-probability sampling.
A more complex version of snowball sampling is called respondent driven sampling. Not only does this approach involve the study participants helping to identify other potential participants, it involves some analysis of the social networks that are tapped into throughout the snowball process. For example, investigators interested in addressing questions related to the experience of human trafficking might begin with identifying a small number of individuals willing to participate in their study (the sample nucleus). These individuals would each identify one or more others who could be invited to participate. Those who accept the invitation and become participants would each identify one or more others. The hard work for study investigators begins with identifying a sufficiently diverse initial core: if they identify too many within the same social network, these members will all be identifying each other or the same few and the snowball will cease to grow. The hard work continues in terms of ensuring protection of privacy rights for those nominating others and for those being nominated for invitation. Then, the investigators must take into consideration the potential bias built into the snowball approach—these people are sufficiently similar to know each other. Entire networks of diverse others may be missed in the snowball process. This limitation is based on the principle of homophily: the observation that people tend to associate with others similar to themselves in ways that are meaningful to them (i.e., race, ethnicity, social class, gender identity, sexual orientation, political or religious belief systems, athletic team loyalty, drinking habits, and many more). The saying “birds of a feather flock together” is true of human social networks, as well.
Participant Recruitment
Successfully engaging sufficient numbers and diversity of participants in a study is critically important to the study’s success. Participant recruitmentcan be conceptualized as a 3-step process (adapted from Begun, Berger, & Otto-Salaj, 2018):
- Generating initial contacts is about identifying and soliciting potential participants. This step is accomplished through a variety of advertising strategies: media advertisements (including social media outlets), posters and flyers, and mass mailings (postal and e-mail). Some success is achieved through telephone calls, as well. In recent years, the rate at which people respond to many of these approaches has declined sharply—people are finding themselves saturated with claims for their attention, and are ignoring many of these messages as “spam” or “junk” mail. Investigators also need to critically consider the potential sources of bias related to different possibilities. For example, individuals’ responsiveness different types of on-line social media is influenced by age group (Snapchat, twitter, and Facebook, for example, are used differently by adolescents, emerging adults, and older adults); reading newspapers is heavily influenced by geography and social class; receiving church newsletter postings is dependent on affiliation; and, reading bus advertisements is dependent on who rides buses and which routes they frequent. In-person recruitment might be effective in specialized locations (e.g., clinics) but may not be effective with the general public (e.g., stores and shopping malls). These, too, are subject to bias in terms of who will agree to listen to the recruitment message and who will not.
Like any advertising effort, the nature of the message is important to consider. The direct contact tool needs to be crafted with consideration of why someone would WANT to engage with the study. The message needs to capture attention first, then needs to capture interest next. It also needs to be easy for someone to respond—a 24/7 phone line or e-mail address or website is more accessible than a phone number with an answering machine. Furthermore, cultural competence of the message needs to be taken into consideration: images and language need to convey a sense that “this study is about people like me.”
- Screening is part of the recruitment process, as well. If strong effort is placed on the first step (generating contacts), there is strong likelihood that individuals who do not meet the study criteria will be contacted. The study investigators need to have clearly defined criteria for study inclusion/exclusion. This process can be as simple or as complex as the criteria for participation. If the study is limited to adults, then the screening can simply be a question: Are you at least 18 years of age? Anyone who answers “no” to this question fails to meet the inclusion criteria and is excluded from participation. Consider the example of a study concerning the relationship of social work education and self-esteem on social work students’ social discrimination of persons with disabilities (Bean, & Hedgpeth, 2014). The direct contact method used in this survey study was to distribute the survey only to individuals who met the study criteria: being in the last semester of their social work program. On the other hand, had they distributed the survey to the entire school, they would have needed to impose a screening strategy to ensure that other students did not become enrolled in the study.
Four difficulties arise in regard to screening. First, investigators need to identify reliable, valid screening measures for each of the study criteria. Second, the screening information cannot be treated as data in the study unless consent was secured for screening and for the main study. Third, investigators need to have an appropriate response for individuals who become excluded. Consider, for example, individuals with a substance use problem who are interested in participating in a treatment study, but who fail to meet the study criteria. Ethically, investigators cannot simply turn them away without offering strong alternatives. Fourth, screening is an important part of the participant-investigator relationship. If the screening experience is tedious or otherwise unpleasant, the chances of retaining participants in the actual study diminish.
- Consenting study participants is a process, not an outcome. A signed consent form simply provides documentation that the process was engaged—the process is the goal. This means that investigators studies need to engage in interactions with potential study participants that ensures they are fully informed about the study and what should be expected—including any potential risk and benefits, as well as steps the investigators take to minimize risk. Making sure of potential participants’ comprehension of the consent information is a responsibility of study investigators. The IRB review process helps ensure that the proposed procedures meet expectations for protecting potential participants rights. In addition to providing participants with sufficient information to make an informed choice about engaging with the study, investigators need to ensure that participants are making the decision without influence of coercion. Some potential coercion scenarios are more obvious than others. For example, instructors who wish to engage their students in a study should not be in the position of knowing who does or does not consent to participate, since students may believe that this knowledge could affect their grade in the course. This is a problem to be addressed in social work intervention research: clients may feel obligated to participate in a study conducted by the person delivering them services, out of concern that the decision might affect the services they receive or out of a desire to help the person who has been helping them. Problems might arise in relation to incentives offered to study participants, too. The amount offered might be considered coercive: $25 may not be coercive to people in some circumstances, but is a lot of money to people in other circumstances, potentially affecting their decision about participation. Legally, children and adolescents cannot provide consent—they have not achieved the age of majority. Thus, persons responsible for ensuring their welfare need to provide consent (parents, legal guardians). At the same time, investigators have a responsibility to secure the child’s/adolescent’s assent to participate. This means that the child or adolescent agrees to the conditions of participation.
Exceptions do exist: sometimes the consent process or consent documentation is waived by an IRB. Sometimes it is not reasonable to secure assent. Consider, for example, studies conducted with infants who would not understand an investigator’s description of what is expected to happen. The investigators need to engage parents/guardians in consent and then observe the infants for cues as to their willingness to continue—for example, crying is a clear sign that the baby is done participating, assent has ended.
Participant Retention
In longitudinal research, it is critical that study participants remain engaged with the study through all phases of data collection. While participant recruitment is about people initially enrolling, participant retention is about them staying involved over time. Obviously, retention is not an issue in cross-sectional research studies. An important point for investigators to keep in mind is that retention begins with recruitment—retention only happens when study participants decide that the experience continues to be worthwhile.
The opposite of participant retention is calledparticipant attrition—people dropping out of a study before completion. The obvious problem with attrition is that the number of participants may drop below the level needed for a strong study design and generalizability from the smaller sample to the population. A less obvious problem is that attrition is seldom a random process. This means that sampling bias may become introduced into the study despite all the care and effort that investigators may have applied in their initial probability random selection. For example, men or persons of color may drop out at a higher rate than women or white participants. The sample at the end of the study is what matters, so minimizing attrition is an important issue for investigators to address. It is costly to replace missing participants—starting over with recruitment and all the data collection efforts that need to be repeated (not to mention paying incentives again). Investigators need to constantly address the question: why would participants WANT to remain involved with the study?
Researchers have demonstrated that the rate of participant attrition tends to be greatest at the start of a study: if someone is going to drop out, this is most probable early on (Begun, Berger, & Otto-Salaj, 2018). That does not mean they will certainly be retained once they are involved in middle and later phases of a study; the dropout rate tends to slow down to more of a trickle as time passes. This means that investigators might engage in their most strenuous, costly retention efforts early on. In addition to making the study protocol interesting and a positive experience for participants, developing good rapport and effective systems for tracking and maintaining contact are critical.
Chapter Summary
This is the final chapter of Module 3. Here you learned about sampling in quantitative research studies, and about participant numbers and heterogeneity (diversity) as important issues in both qualitative and quantitative research. This chapter also discussed aspects of participant recruitment and retention that are important in both qualitative and quantitative studies.
While it is not quite the same as recruiting you to participate in a research study, let’s think about how the content of this chapter relates to your participation in end-of-semester course/instructor evaluations. For many courses, the response rate is 30% or less—meaning that 2/3 of your classmates do not participate.
What is the greatest potential bias risk to the program’s evaluation results of failing to recruit participants like you (and many of your peers)?
In a class of 25 students, how many do you think would be a good number to recruit for a strong sample?
What are 3-5 things the program could do to increase your participation in the evaluation process—what makes you WANT to participate?
Does reading this chapter and thinking about this topic make the likelihood of your participation in evaluating this course greater, less, or about the same? Why?